Marine Robotics Datasets Australian Centre for Field Robotics
Tasmania Coral Point Count (ACFR/UTas)
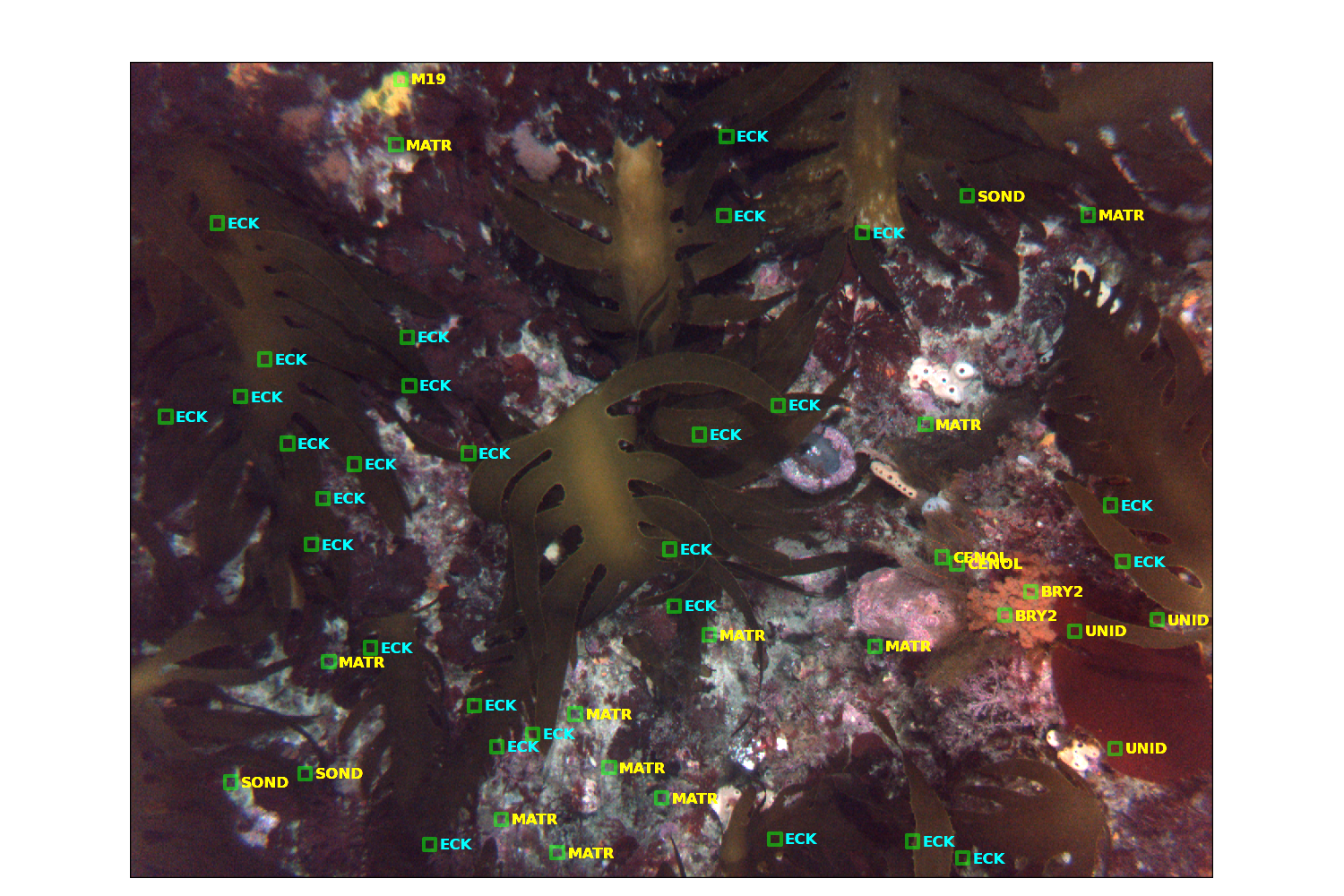
Example image with labels
Description:
The data set is comprised of 22 dive missions conducted by AUV Sirius off the South-East coast of Tasmania in October 2008. From the data set containing over 100,000 stereo pairs of images, marine scientists selected every 100th colour image, and used the CPCe software package to label 50 random points on each. Figure 1 shows an example image containing the expert annotations.
A wide range of class labels were used, indicating biological species (including types of sponge, coral, algae and others), abiotic elements (types of sand, gravel, rock, shells etc.), and types of unknown data (ambiguous species, poor image quality, etc.). Precise details of the labelling methodology can be found in [1]. A simplified version of the labeling hierarchy is shown in Figure 2.
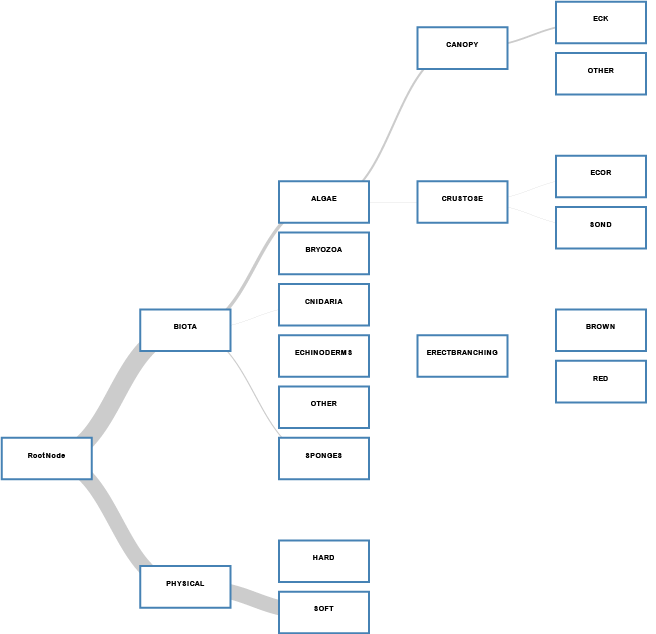
Label hierarchy
Publications
- [1]
- N.S. Barrett, L. Meyer, N. Hill, and P.H. Walsh. Methods for the processing and scoring of AUV digital imagery from south eastern tasmania, Technical Report 11560, University of Tasmania, August 2011.
- [2]
- M.S. Bewley, B. Douillard, N. Nourani-Vatani, A. Friedman, O.R. Pizarro, S.B. Williams, Automated species detection: An experimental approach to kelp detection from sea-floor AUV images, Australasian Conference on Robotics and Automation (ACRA), Dec 2012, New Zealand
- [3]
- M.S. Bewley, N. Nourani-Vatani, B. Douillard, O. Pizarro, S.B. Williams, Hierarchical Classification of Sea-Floor Imagery, CVPR Workshop on Fine Grained Visual Categorization, June 2013, USA
Acknowledgement
We would appreciate if you can send an email to let us know when you use this data set or when the data set appears in a publicaton. Please also include the following acknowledgement:
"The authors would like to acknowledge the Australian National Research Program (NERP) Marine Biodiversity Hub for the taxonomical labeling and the Australian Centre for Field Robotics for gathering the image data".
Download
The following information will soon available for download:
- A README file.
- 1258 color images from the left stereo pair.
- 1258 monochrome images from the right stereo pair.
- CSV file containing the image meta data including labels, 6DOF camera pose, time stamp etc.
- Python scripts to generate the labeling hierarcy.
Scott Reef 25 Scott Reef dense grid, 2009
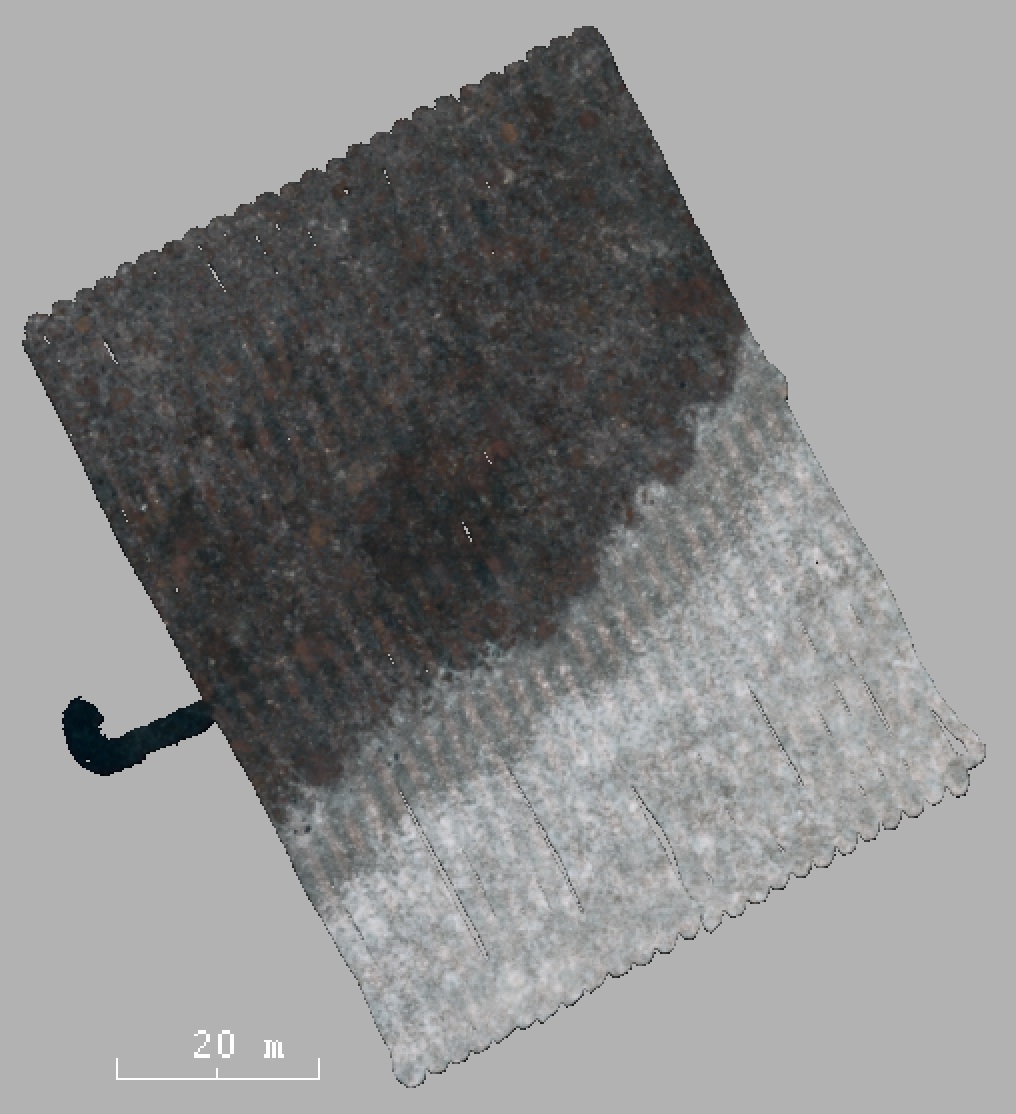
Scott Reef mosaic
9800 stereo image pairs captured by the Sirius AUV densely covering an area of 75mx50m.
Description:
The trajectory consists of 50 parallel tracks and one perpendicular path across the center of the path. This data set has therefore many small loop closures. As can be seen from the mosaic there are three dominating substrates: reef, sand and transition area.
Publications
- [1]
- D. M. Steinberg, O. Pizarro, S.B. Williams, and M.V. Jakuba, Towards autonomous habitat classification using Gaussian Mixture Models, IEEE/RSJ International Conference on Intelligent Robots and Systems (IROS), 2010.
- [2]
- D. M. Steinberg, O. Pizarro, S.B. Williams, and M.V. Jakuba, Dirichlet process mixture models for autonomous habitat classification IEEE OCEANS, 2010
- [3]
- D. M. Steinberg, A. Friedman, O. Pizarro, and S. B. Williams, A Bayesian nonparametric approach to clustering data from underwater robotic surveys, International Symposium on Robotics Research, Flagstaff, AZ, Aug. 2011.
- [4]
- M. Bryson, M. Johnson-Roberson, O. Pizarro and S. Williams, Automated Registration for Multi-year Robotic Surveys of Marine Benthic Habitats, To appear at IEEE/RSJ International Conference on Intelligent Robots and Systems (IROS), Tokyo, Japan, November 2013.
Acknowledgement
We would appreciate if you can send an email to let us know when you use this data set or when the data set appears in a publicaton. Please also include the following acknowledgement:
"The authors would like to acknowledge the Australian Centre for Field Robotics' marine robotics group for providing the data".
Download
- 9831 stereo image pairs [22GB!].
- Multibeam data from the onboard Imagenex DeltaT 260kHz sensor in GSF format [6.2MB].
- Our SLAM solution can form as ground truth or baseline. Also provided is a KML file of the trajectory [1.4MB].
- Raw sensor data from the onboard sensors. These are ASCII files where each line contains a message from a sensor [7.0MB].
Tasmania O'Hara 7 O'Hara Marine Protected Area, Tasmania, 2008
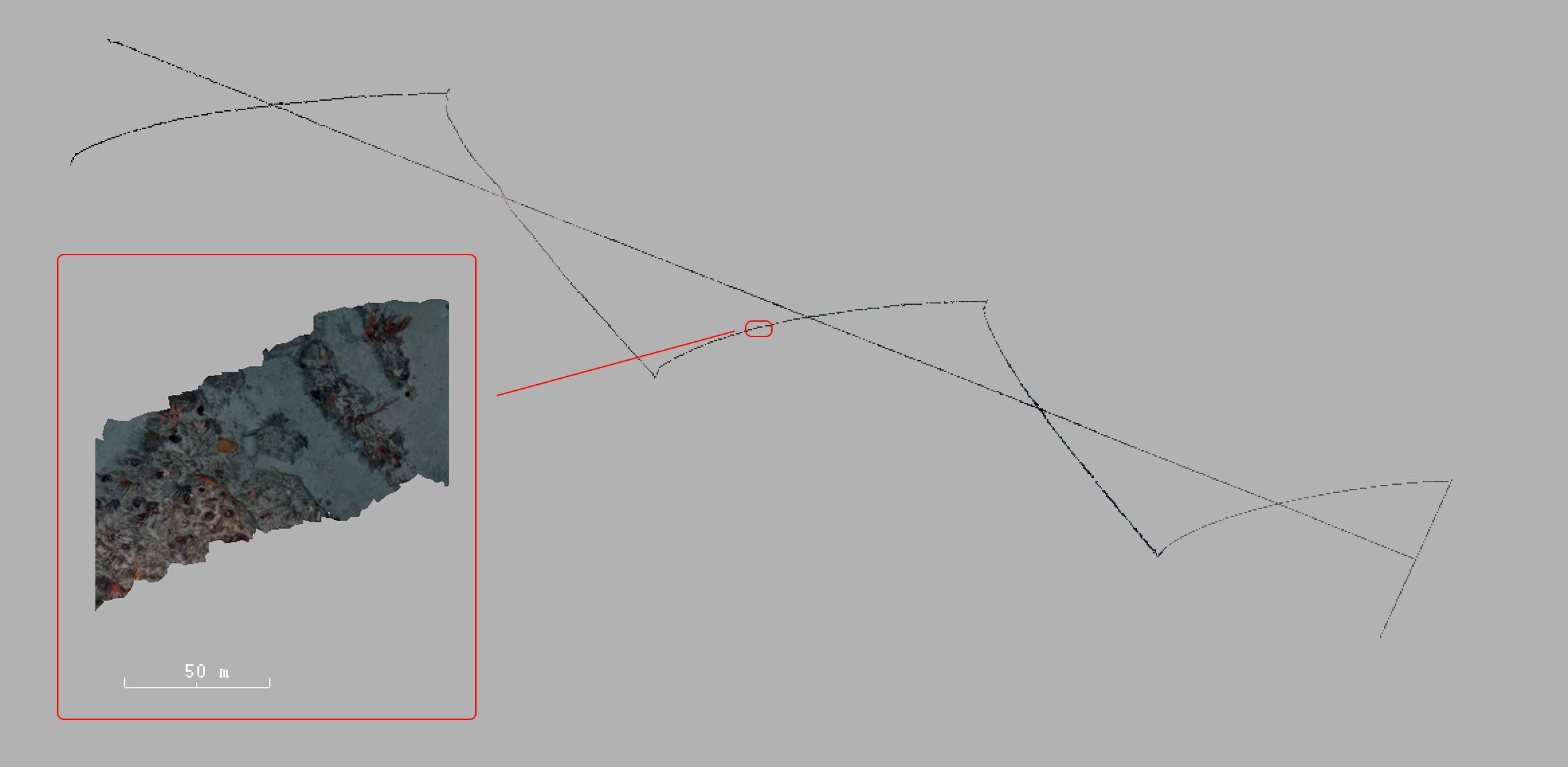
O'Hara reef mosaic
11200 stereo image pairs captured by the Sirius AUV traversing a transect of >4km.
Description:
The trajectory consists of a long zig-zag path and a straight path crossing through it generating five loop closure opportunities. This data set has few but very long loop closures.
Publications
- [1]
- D. M. Steinberg, A. Friedman, O. Pizarro, and S. B. Williams, A Bayesian nonparametric approach to clustering data from underwater robotic surveys, In International Symposium on Robotics Research, Flagstaff, AZ, Aug. 2011.
- [2]
- A. Friedman, D. M. Steinberg, O. Pizarro, and S.B. Williams, Active learning using a Variational Dirichlet Process model for pre-clustering and classification of underwater stereo imagery, IEEE/RSJ International Conference on Intelligent Robots and Systems (IROS), 2011.
- [3]
- M. Bryson, M. Johnson-Roberson, O. Pizarro and S. Williams, Colour-Consistent Structure-from-Motion Models using Underwater Imagery, Robotics: Science and Systems (RSS), Sydney, Australia, July 2012.
- [4]
- A. Bender, S. B. Williams, O. Pizzaro, Classification with probabilistic targets, IEEE/RSJ International Conference on Intelligent Robots and Systems (IROS), 2012.
- [5]
- A. Bender, S. B. Williams, O. Pizzaro, Autonomous exploration of large-scale benthic environments, IEEE International Conference on Robotics and Automation (ICRA), 2013.
Acknowledgement
We would appreciate if you can send an email to let us know when you use this data set or when the data set appears in a publicaton. Please also include the following acknowledgement:
"The authors would like to acknowledge the Australian Centre for Field Robotics' marine robotics group for providing the data".
Download
- 11278 stereo image pairs [24GB!].
- Multibeam data from the onboard Imagenex DeltaT 260kHz sensor in GSF format [60MB].
- Our SLAM solution can form as ground truth or baseline. Also provided is a KML file of the trajectory [1.4MB].
- Raw sensor data from the onboard sensors. These are ASCII files where each line contains a message from a sensor [8.6MB].